Sergey Nikolenko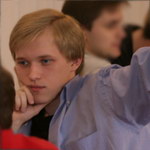 Main page Books Research papers Talks and posters Students Popular science Other stuff Research CS and crypto Bioinformatics Machine learning Algebraic geometry Algebra Bayesian networks Earth sciences Teaching 2014 ML, KFU Game Theory, HSE Mech. Design, HSE ML, CSClub Kazan Game theory, HSE Math. logic, AU Machine learning, STC Machine learning, AU 2013 Discrete math, HSE Machine learning, STC Math. logic, AU Cryptography, AU 2012 Machine learning, STC Math. logic, AU Machine learning II, AU Machine learning, AU Machine learning, EMC 2011 Cryptography, AU Math. logic, AU Machine learning, AU 2010 Math. logic, AU Machine learning, AU Cryptography, AU 2009 Crypto in CS Club Statistics Machine learning, AU Cryptography 2008 Speech recognition MD for CS Club ML for CS Club Mechanism design 2007 Machine Learning Probabilistic learning External links Google Scholar profile DBLP profile LiveJournal account
nikolenko (in Russian) |  |
Teaching activities |
Machine Learning for CS Club: Kazan 2014
This is a short introduction to machine learning presented as part of the
Computer Science Club program
in Kazan; see also the course page.
The course itself (all slides and lecture notes are in Russian):
- 1. Introduction. History of AI. Overview of different problem settings in machine learning.
Probability theory basics. Bayes theorem and maximal a posteriori hypotheses.
- Slides (.pdf, 297kb)
- 2. Sample application of Bayesian ideas: Laplace's rule of succession. Priors. Conjugate priors.
Beta distribution as a conjugate prior for Bernoulli trials. Parametric and nonparametric models: nearest neighbors.
Curse of dimensionality.
- Slides (.pdf, 714kb)
- 3. Linear regression. Least squares, polynomial curve fitting. Overfitting: ridge regression. Ridge regression as Gaussian priors.
Other kinds of regularizers: lasso regression. Linear classification: logistic regression.
- Slides (.pdf, 1673kb)
- 4. Graphical models. Directed graphical models, d-separation. Undirected graphical models. Factor graphs.
Message passing: sum-product on a chain, sum-product on a general tree. Overview of approximate inference algorithms.
- Slides (.pdf, 1504kb)
- 5. The Expectation-Maximization algorithm: mixture of Gaussians for clustering, general case. Hidden Markov models: definitions, the three problems, the Baum--Welch algorithm.
- Slides (.pdf, 710kb)
- 6. Sample applications of probabilistic modeling: text categorization (naive Bayes), topic modeling (LDA), recommender systems (nearest neighbors and SVD), Bayesian rating systems (TrueSkill).
- Slides (.pdf, 3391kb)
Selected references.
- Christopher M. Bishop. Pattern Recognition and Machine Learning. Springer, Information Science and Statistics series, 2006.
- Kevin Murphy. Machine Learning: A Probabilistic Perspective, MIT Press, 2012.
- David J. C. MacKay. Information Theory, Inference, and Learning Algorithms. Cambridge University Press, 2003.
|