Sergey Nikolenko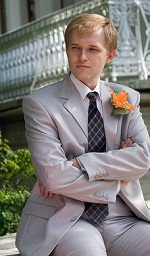 Main page
print ' Books';
print ' Research papers';
print ' Talks and posters';
print ' Students';
print ' Popular science';
print ' Other stuff';
print ' Research';
print ' CS and crypto';
print ' Bioinformatics';
print ' Machine learning';
print ' Algebraic geometry';
print ' Algebra';
print ' Bayesian networks';
print ' Earth sciences';
print ' Teaching';
print ' 2014';
print ' ML, KFU';
print ' Game Theory, HSE';
print ' Mech. Design, HSE';
print ' ML, CSClub Kazan';
print ' Game theory, HSE';
print ' Math. logic, AU';
print ' Machine learning, STC';
print ' Machine learning, AU';
print ' 2013';
print ' Discrete math, HSE';
print ' Machine learning, STC';
print ' Math. logic, AU';
print ' Cryptography, AU';
print ' 2012';
print ' Machine learning, STC';
print ' Math. logic, AU';
print ' Machine learning II, AU';
print ' Machine learning, AU';
print ' Machine learning, EMC';
print ' 2011';
print ' Cryptography, AU';
print ' Math. logic, AU';
print ' Machine learning, AU';
print ' 2010';
print ' Math. logic, AU';
print ' Machine learning, AU';
print ' Cryptography, AU';
print ' 2009';
print ' Crypto in CS Club';
print ' Statistics';
print ' Machine learning, AU';
print ' Cryptography';
print ' 2008';
print ' Speech recognition';
print ' MD for CS Club';
print ' ML for CS Club';
print ' Mechanism design';
print ' 2007';
print ' Machine Learning';
print ' Probabilistic learning';
print ' External links';
print ' Google Scholar profile';
print ' DBLP profile';
print ' LiveJournal account
nikolenko (in Russian) | ';
print ' | ';
?>
Teaching activities |
Automated Speech Recognition
Fall of 2008, SPSU IFMO.
An introductory course on speech recognition, covering DSP basics, MFCC features and feature selection, hidden Markov models, ANNs and TD-ANNs, and language modeling.
A sample TeX file for the lecture notes (lecture notes for lecture 2).
Another sample TeX file (sample from lecture 9, with figures and examples).
The course itself (all slides and lecture notes are in Russian):
- 1. Introduction. ASR challenges and course plan.
- 2. Signals and systems. Convolution. Integrators and differentiators. The Dirac delta function.
- Lecture notes by Oleg Dahin (.pdf, 165kb)
- 3. Eigenfunctions. Fourier series. Plancherel theorem. Periodic convolution. Discrete Fourier series. Fourier transform. Filters and filter banks.
- Lecture notes by Andrey Borisenko and Alexander Koshevoy (.pdf, 201kb)
- 4. Kotelnikov (Nyquist-Shannon) theorem. Examples of Fourier transforms. Fast Fourier transform. Filters and rational functions. FIR and IIR filters. Windows.
- 5. Speech signals. Spectrograms. Linear predictive coding. Autocorrelations. The Levinson-Durbin algorithm. Cepstrum.
- 6. Features of a speech signal. Filter banks, LPC, mel cepstrum. Discrete cosine transform. Mel scale. MFCC.
- Lecture notes by Eugene Selifonov and Andrey Tikhomirov (.pdf, 213kb)
- 7. Feature selection. Principal components analysis. Minimizing error.
- Lecture notes by Sergey Gindin and Andrey Davydov (.pdf, 202kb)
- 8. The kernel trick. Kernel PCA. Commonly used kernels. Kernel k-means clustering.
- Lecture notes by Catherine Vasilyeva and Egor Smirnov (.pdf, 179kb)
- 9. Hidden Markov models. Dynamic programming, Viterbi algorithm. The Baum-Welch algorithm. Kullback-Leibler distance.
- Slides ()
Lecture notes by Sergey Nikolenko ()
- 10. Special cases of HMMs. Continuous distributions. Autoregressive HMMs. Optimization criteria: ML, MMI, MDI.
- Slides ()
- 11. Artificial neural networks. Backpropagation.
- Lecture notes by Daniel Penkin (.pdf, 192kb)
- 12. Time-delay ANNs (TD-ANN). Temporal backpropagation.
- 13. Language modeling. Grammars, context-free grammars, Chomsky normal form. Chart parser. Probabilistic context-free grammars.
- Lecture notes by Jan Malakhovski (.pdf, 210kb)
- 14. Language modeling. N-grams. Cross-entropy and perplexity. N-gram smoothing: backoff models, interpolation, Kneser-Ney smoothing.
Selected references.
- Xuedong Huang, Alex Acero, Hsiao-Wuen Hon. Spoken language processing: a guide to theory, algorithm, and system development. Prentice Hall, 2001.
|