Sergey Nikolenko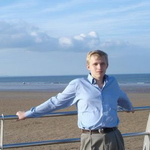 Main page
print ' Books';
print ' Research papers';
print ' Talks and posters';
print ' Students';
print ' Popular science';
print ' Other stuff';
print ' Research';
print ' CS and crypto';
print ' Bioinformatics';
print ' Machine learning';
print ' Algebraic geometry';
print ' Algebra';
print ' Bayesian networks';
print ' Earth sciences';
print ' Teaching';
print ' 2014';
print ' ML, KFU';
print ' Game Theory, HSE';
print ' Mech. Design, HSE';
print ' ML, CSClub Kazan';
print ' Game theory, HSE';
print ' Math. logic, AU';
print ' Machine learning, STC';
print ' Machine learning, AU';
print ' 2013';
print ' Discrete math, HSE';
print ' Machine learning, STC';
print ' Math. logic, AU';
print ' Cryptography, AU';
print ' 2012';
print ' Machine learning, STC';
print ' Math. logic, AU';
print ' Machine learning II, AU';
print ' Machine learning, AU';
print ' Machine learning, EMC';
print ' 2011';
print ' Cryptography, AU';
print ' Math. logic, AU';
print ' Machine learning, AU';
print ' 2010';
print ' Math. logic, AU';
print ' Machine learning, AU';
print ' Cryptography, AU';
print ' 2009';
print ' Crypto in CS Club';
print ' Statistics';
print ' Machine learning, AU';
print ' Cryptography';
print ' 2008';
print ' Speech recognition';
print ' MD for CS Club';
print ' ML for CS Club';
print ' Mechanism design';
print ' 2007';
print ' Machine Learning';
print ' Probabilistic learning';
print ' External links';
print ' Google Scholar profile';
print ' DBLP profile';
print ' LiveJournal account
nikolenko (in Russian) | ';
print ' | ';
?>
Teaching activities |
Fundamentals of mathematical statistics
This course is presented in the «Academic University of Physics and Technology»
as part of the recently established Chair of Mathematics and Computer Science.
The course itself:
- 1. Introduction. Probability distributions: binomial, normal, exponential, Poisson. Law of large numbers and the central limit theorem.
- 2. Maximum likelihood estimators. Properties. Method of moments. Biased and unbiased estimators. Asymptotic normality.
- 3. Fischer information. Convergence and asymptotic normality of the MLE. Examples.
- 4. The Rao-Cramer inequality. Efficient estimators.
- 5. Prior and posterior distributions. Conjugate priors. Examples: Bernoulli trials, Poisson distribution. Equivalent sample size.
- 6. Sufficient statistics. The Neyman-Fischer factorization criterion.
- 7. Hypotheses testing. Simple hypotheses. False positives and false negatives. Bayesian decision rules. Examples.
- 8. The most powerful test for simple hypotheses. Likelihood ratios. One-sided hypotheses. Most powerful test for a one-sided hypothesis.
- 9. The Pearson theorem. Covariance.
- 10. Chi-square tests. Examples. Composite hypotheses and chi-square tests for them. Independence and homogeneity tests.
- 11. Fischer distribution. Student's t-distribution. Confidence intervals for the normal distribution parameters.
- 12. The Kolmogorov-Smirnov test.
- 13. Linear regression. Least squares, statistical justification. Confidence intervals on the linear regression parameters.
|