Sergey Nikolenko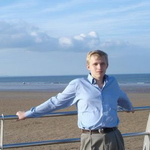 Main page Books Research papers Talks and posters Students Popular science Other stuff Research CS and crypto Bioinformatics Machine learning Algebraic geometry Algebra Bayesian networks Earth sciences Teaching 2014 ML, KFU Game Theory, HSE Mech. Design, HSE ML, CSClub Kazan Game theory, HSE Math. logic, AU Machine learning, STC Machine learning, AU 2013 Discrete math, HSE Machine learning, STC Math. logic, AU Cryptography, AU 2012 Machine learning, STC Math. logic, AU Machine learning II, AU Machine learning, AU Machine learning, EMC 2011 Cryptography, AU Math. logic, AU Machine learning, AU 2010 Math. logic, AU Machine learning, AU Cryptography, AU 2009 Crypto in CS Club Statistics Machine learning, AU Cryptography 2008 Speech recognition MD for CS Club ML for CS Club Mechanism design 2007 Machine Learning Probabilistic learning External links Google Scholar profile DBLP profile LiveJournal account
nikolenko (in Russian) |  |
Teaching activities |
Machine Learning for CS Club
This course unites «Machine Learning» and
«Probabilistic learning» and aims to provide a
comprehensive review of machine learning (mainly from the Bayesian perspective) in a
relatively short course.
This course is presented in the «Computer Science Club»
in the Steklov Mathematical Institute supported by Anton Likhodedov and run by
Edward A. Hirsch and Alexander Kulikov.
The course itself (all slides and lecture notes are in Russian):
- 1. Introduction. History of AI. Probability theory basics. Bayes theorem and maximal a posteriori hypotheses. A review of useful distributions.
- Slides (.pdf, 1077kb)
- 2. Decision trees. ID3. Bayesian analysis of classification problems. Complexity measures based on decision trees and bounds on decision tree complexity.
- Slides (.pdf, 1056kb)
- 3. Artificial Neural Networks. Perceptrons; learning a linear perceptron. Non-linear perceptrons and gradient descent. ANNs and the backpropagation algorithm. Modifications: momenta, regularizers.
- Slides (.pdf, 999kb)
- 4. Sampling. The sampling problem, why it is hard. Importance sampling and rejection sampling. MCMC (Monte-Carlo Markov Chain) methods. The Metropolis-Hastings method, Markov chains, slice sampling. Hamiltonian mechanics and Hamiltonian MCMC.
- Slides (.pdf, 652kb)
- 5. Genetic algorithms. Crossover on binary strings and trees. Genetic programming. Baldwin effect. Ant Colony optimization. Simulated annealing.
- Slides (.pdf, 1384kb)
- 6. Bayesian classifiers: optimal, Gibbs, and naive. Concept learning: Find-S and Candidate Elimination algorithms.
- Slides (.pdf, 622kb)
- 7. Bayesian Belief Networks. Definitions, d-separability, decomposition. Propagation in polytree BBNs. Propagation in BBNs with undirected cycles: join and junction trees, triangulation, message passing algorithm.
- 8. Brute force marginalization. Conjugate priors. Some coding theory: error-correcting codes, codes and their trellises. Decoding as Bayesian inference. Sum-product and min-sum algorithms.
- Slides (.pdf, 947kb)
- 9. Marginalization on a graph. Sum-product and min-sum in the general case. Approximate marginalization: Laplace method. Marginalization with Kikuchi approximation methods.
- Slides (.pdf, 702kb)
- 10. Hidden Markov models. The model, three inference tasks. The Viterbi, sum-product, and Baum-Welch algorithms.
- Slides (.pdf, 720kb)
- 11. Associative memory. Hebbian learning. Bidirectional associative memory. Hopfield networks and a proof of their convergence.
- Slides (.pdf, 461kb)
Selected references.
- A.L. Tulupyev, S.I. Nikolenko, A.V. Sirotkin. Bayesian Networks: A Probabilistic Logic Approach. St.-Petersburg, Nauka, 2006. (two first pages of the book: .pdf, 815kb, in Russian, ozon.ru)
- David J. C. MacKay. Information Theory, Inference, and Learning Algorithms. Cambridge University Press, 2003.
|